Duration
Description
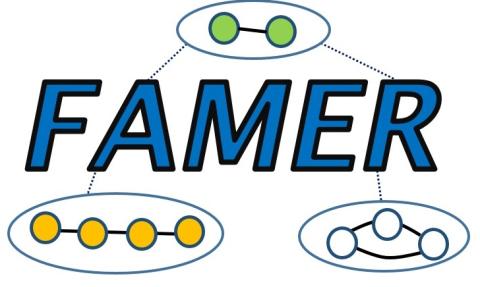
FAMER (FAst Multi-source Entity Resolution system) is a scalable framework for distributed multi-source entity resolution. It can construct similarity graphs for entities of multiple sources based on different linking schemes; existing links from the Web of Data could also be used to build the similarity graph. FAMER also provides several entity clustering schemes. They use the similarity graph to determine groups of matching entities aiming at maximizing the similarity between entities within a cluster and minimizing the similarity between entities of different clusters. Moreover, FAMER is able to repair clusters, e.g. that are overlapping and/or source-inconsistent.
FAMER is also able to perform the incremental matching process. The approach uses a so-called clustered similarity graph, i.e., a similarity graph reflecting already determined clusters. The input of the workflow is a stream of new entities from existing sources or from a new source plus the already determined clustered similarity graph from previous iterations. The incremental Linking and Clustering/Repairing part supports two general approaches for integrating the group of new entities into clusters. In the base (non-repairing) approach the new entities are either added to a similar existing cluster or they form a new cluster. A more sophisticated approach is able to repair existing clusters to achieve a better cluster assignment for new entities by reclustering a portion of the existing clustered graph. The output of incremental clustering is a fully clustered graph. The clusters can optionally be fused in the Fusion component so that all entities are represented by a single entity called cluster representative.
FAMER is implemented using Apache Flink™ so that the calculation of similarity graphs and the clustering approaches can be executed in parallel on clusters of variable size. We have also developed a visualization tool, SIMG-Viz to visually analyze the similarity graphs and clusters determined by FAMER.
Main Objectives:
- Efficient parallel execution of match workflows in the cloud
- Efficient application of clustering schemes for entity matching
- Efficient methods for entity matching repairing
- Efficient parallel execution for incremental linking and clustering.
Initial release and documentation:
We provided an initial release and documentation of FAMER as well as a test dataset for a entity resolution/clustering challenge at the EDBT 2019 summer school on data integration. It is also a good starting point for using FAMER in other projects.
Source Code
Funding / Cooperation
This work is partially funded by the German Federal Ministry of Education and Research under project ScaDS Dresden/Leipzig (BMBF 01IS14014B).
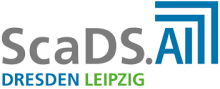
Publikationen (9)
Dateien | Cover | Beschreibung | Jahr |
---|---|---|---|
![]() |
2021 / 10 | ||
![]() |
2021 / 3 | ||
![]() |
2020 / 6 | ||
![]() |
Obraczka, D.
; Saeedi, A.
; Rahm, E.
Proc. KDD workshop on Data Integration to Knowledge Graphs (DI2KG) (DI2KG Challenge Winner)
|
2019 / 8 | |
![]() |
Nentwig, M.
; Rahm, E.
Proc. IEEE International Conference on Data Mining Workshop, ICDMW 2018, Singapore
|
2018 / 11 | |
![]() |
Saeedi, A.
; Nentwig, M.
; Peukert, E.
; Rahm, E.
Complex Systems Informatics and Modeling Quarterly (CSIMQ), Issue 16, Sep./Oct. 2018, pp 61–83
|
2018 / 11 | |
![]() |
2018 / 6 | ||
![]() |
2018 / 3 | ||
![]() |
2017 / 9 |