Duration
Description
Overview
Record linkage aims at linking records that refer to the same real-world entity, such as persons. Typically there is a lack of global identifiers, therefore the linkage can only be achieved by comparing available quasi-identifiers, such as name, address or date of birth. However, in many cases, data owners are only willing or allowed to provide their data for such data integration if there is sufficient protection of sensitive information to ensure the privacy of persons, such as patients or customers. For example, in medical research data of several sources (e. g., hospitals) has to be matched to investigate possible correlations between some diseases of the same patients without revealing the identity of patients.
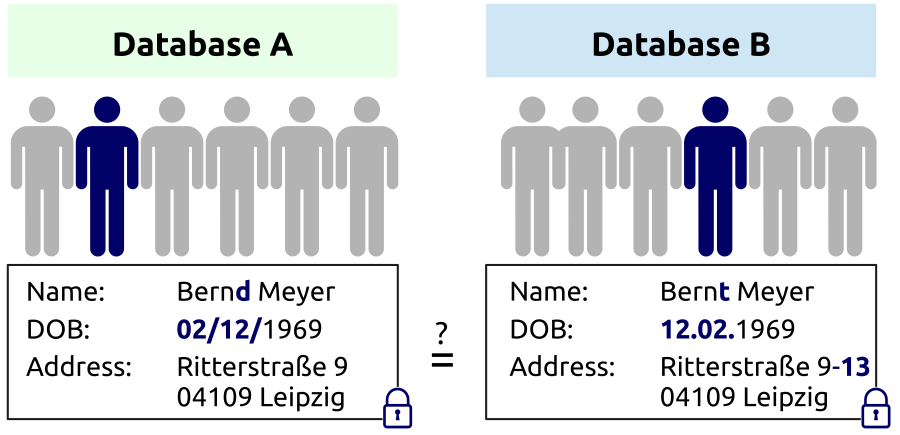
Privacy Preserving Record Linkage (PPRL) addresses this problem by providing techniques to match records while preserving their privacy allowing the combination of data from different sources for improved data analysis and research. For this purpose, the linkage of person-related records is based on encoded values of the quasi-identifiers and the data needed for analysis (e. g., health data) is separated from these quasi-identifiers. The relevant data can be provided to a researcher without the identifying data. Those PPRL approaches can be applied in many areas, such as public health surveillance, demographical studies and marketing analysis. PPRL is confronted with several challenges needing to be solved to ensure its practical applicability. In particular, a high degree of privacy has to be ensured by suitable encoding of sensitive data and organizational structures, such as the use of a trusted linkage unit. PPRL must achieve a high linkage quality by avoiding false or missing matches. Furthermore, a high efficiency with fast linkage time and scalability to large data volumes are needed. A main problem for performance is the inherent quadratic complexity of the linkage problem when every record of the first source is compared with every record of the second source. For better efficiency, the number of comparisons can be reduced by adopting blocking or filtering approaches. Furthermore, the matching can be performed in parallel on multiple processing nodes.
Research Results
In the last few years, we concentrated on improving the performance and scalability of our PPRL methods. As the records are represented as bit vectors (using Bloom filters for encoding), we used metric space similarity measures for filtering. In particular, the pivot-based approach for metric spaces utilizing the triangle inequality to reduce the search space showed significant improvement of performance compared to previous filter techniques. One data source is indexed by determining some records as pivots and assigning the leftover records to them. We can save many similarity computations by comparing the records of the second source with only the pivots first and exclude most records as possible matches.
Recently, we parallelized this approach utilizing the modern framework Apache Flink, which supports efficient parallel and distributed in-memory processing. We developed parallel algorithms for both determining the pivots and the pivot-based matching process for different strategies to select the pivots. The distributed implementation outperforms the centralized version for large dataset and improves the scalability of this approach.
Additionally, we investigated locality-sensitive hashing (LSH) as blocking method and also developed a distributed algorithm using Apache Flink. LSH enables probabilistic blocking by applying hash functions on bit vectors (Bloom filters). LSH-based blocking supports a flexible configuration and can be applied on encoded data. An extensive evaluation has shown that LSH-based blocking can achieve high quality and high scalability up to millions of records. In contrast, phonetic blocking approaches based on Soundex are susceptible to cryptanalysis and more sensitive with respect to data quality problems. Moreover, phonetic blocking based on a single attribute is not feasible for large datasets due to the low number of blocks and data skew effect introduced by frequent names.
Furthermore, we analyzed methods for multi-party PPRL considering applications, where more than two data holder are involved in the process of privacy preserving record linkage. The most challenging case of multiparty PPRL is to find subset matches, i. e. sets of matching records that are not in all sources, but in subsets of them. The intuitive way is to link the sources sequentially using for example the metric space as a growing index structure. In each iteration a new source is linked with the other already matched sources, then indexed. At the end of this process resulting clusters need to be cleaned if we consider that the sources are deduplicated (only one records from each source). Solutions to this problem are still a research topic.
Students
- Caroline Mösler
- Thomas Hoppe
- Lennart Laverenz
- Max Schrodt
- Lukas Burges
- Elias Messner
- Duc Dung Dao
- Manuel Friedrich
- Tim Häntschel
- Manh Le Dac
- Malek Bakleh
- Theresa Butenschön
- Markus Wetzel
- Bennet Friedrichs
Publikationen (20)
Dateien | Cover | Beschreibung | Jahr |
---|---|---|---|
![]() |
2025 / 6 | ||
![]() |
Rohde, F.
; Christen, V.
; Franke, M.
; Rahm, E.
21st Conference on Database Systems for Business, Technology and Web (BTW 2025)
|
2025 / 3 | |
![]() |
Franke, M.
Universität Leipzig
|
2024 / 5 | |
![]() |
Franke, M.
; Christen, V.
; Christen, P.
; Rohde, F.
; Rahm, E.
27th International Conference on Extending Database Technology
|
2024 / 3 | |
![]() |
Intemann, T.
; Kaulke, K.
; Kipker, D.
; Lettieri, V.
; Stallmann, C.
; Schmidt, C.
; Geidel, L.
; Bialke, M.
; Hampf, C.
; Stahl, D.
; Lablans, M.
; Rohde, F.
; Franke, M.
; Kraywinkel, K.
; Kieschke, J.
; Bartholomäusn, S.
; Näher, A.
; Tremper, G.
; Lambarki, M.
; March, S.
; Prasser, F.
; Haber, A. C.
; Drepper, J.
; Schlünder, I.
; Kirsten, T.
; Pigeot, I.
; Sax, U.
; Buchner, B.
; Ahrens, W.
; Semler, S.
Fachrepositorium Lebenswissenschaften
|
2023 / 8 | |
![]() |
Rohde, F.
; Franke, M.
; Christen, V.
; Rahm, E.
Conference on Database Systems for Business, Technology and Web (BTW) 2023
|
2023 / 3 | |
![]() |
Christen, V.
; Häntschel, T.
; Christen, P.
; Rahm, E.
International Journal of Data Science and Analytics
|
2022 / 12 | |
![]() |
Franke, M.
; Sehili, Z.
; Rohde, F.
; Rahm, E.
Proceedings of the 24th International Conference on Extending Database Technology (EDBT), pp. 289-300
|
2021 / 3 | |
![]() |
Sehili, Z.
; Rohde, F.
; Franke, M.
; Rahm, E.
Proc. 19. GI-Fachtagung für Datenbanksysteme für Business, Technologie und Web (BTW), 2021
|
2021 / 2 | |
![]() |
2021 / 1 |